Retrieval-Augmented Generation (RAG) has revolutionized the way we interact with information. By combining the power of search and generative AI, RAG systems can provide comprehensive and informative responses to complex queries. However, traditional RAG models primarily focus on textual data, limiting their ability to understand and process the richness of the real world.
Multi-modal RAG (MM-RAG) addresses this limitation by incorporating multiple data modalities, such as images, videos, and audio, into the RAG pipeline. This groundbreaking approach empowers AI systems to comprehend and generate responses based on a wider range of information, leading to more accurate, informative, and engaging outputs.
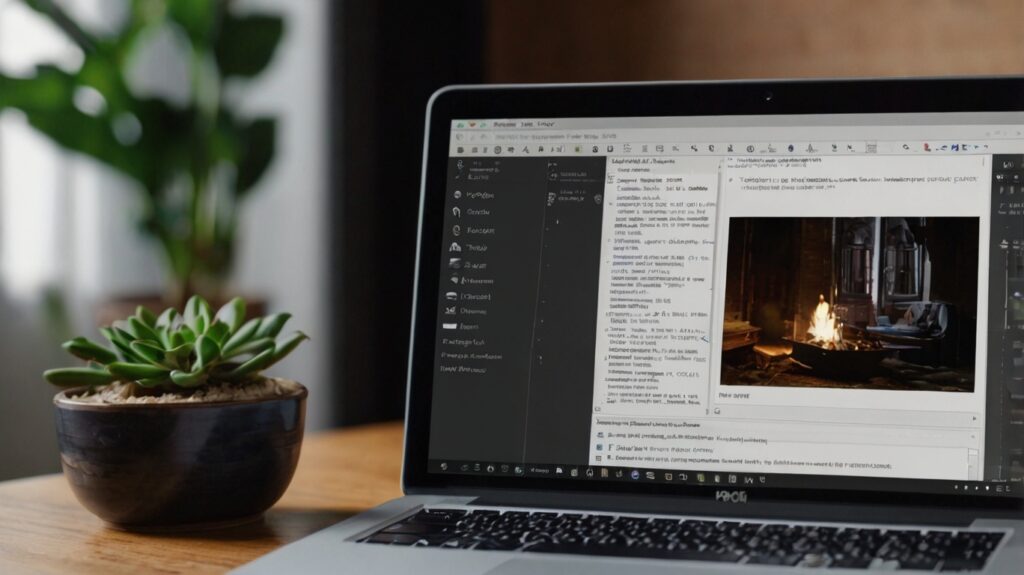
In this blog post, we will delve into the latest advancements in MM-RAG, exploring different approaches, their underlying structures, and the advantages they offer over traditional methods. We will also discuss the challenges and limitations of these new approaches.
Table of Contents
ToggleUnderstanding Multi-Modal RAG
Before diving into the latest approaches, let’s briefly recap the core components of a traditional RAG system:
- Retrieval: Relevant information is extracted from a knowledge base based on the query.
- Generation: A language model generates a response using the retrieved information.
In MM-RAG, the retrieval component is extended to handle multiple data modalities. This involves:
- Multimodal Embedding: Converting different data types (text, images, videos, etc.) into a common vector space.
- Multimodal Search: Efficiently searching through the multimodal knowledge base to retrieve relevant information.
The generation component is also enhanced to process and integrate information from various modalities, requiring more sophisticated language models capable of handling multimodal inputs.
Latest Approaches in Multi-Modal RAG
1. Fusion-Based Approaches
Fusion-based approaches combine information from different modalities at different stages of the RAG pipeline. This can be achieved through:
- Early Fusion: Combining modalities at the embedding level, creating a unified representation for all data points.
- Late Fusion: Processing each modality separately and combining the results at the generation stage.
- Hybrid Fusion: A combination of early and late fusion, allowing for flexibility in handling different data types.
Advantages:
- Can effectively capture complex relationships between modalities.
- Offers flexibility in combining different data types.
Limitations:
- Requires careful design of fusion mechanisms.
- May suffer from information loss due to early fusion.
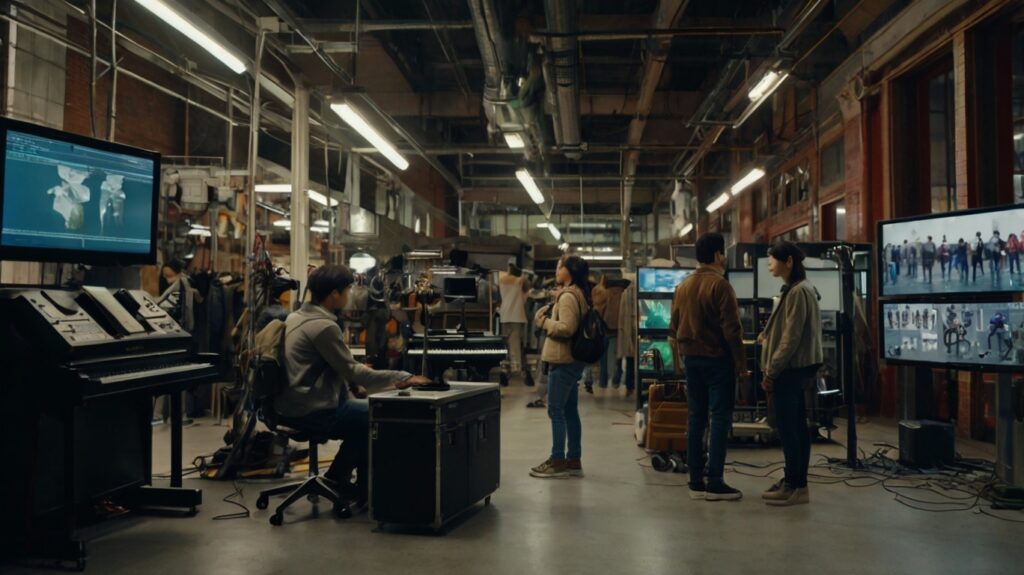
2. Attention-Based Approaches
Attention mechanisms have proven to be highly effective in capturing dependencies between different parts of a sequence. In MM-RAG, attention can be used to:
- Attend to relevant parts of different modalities: Focusing on the most informative regions of images or videos.
- Align information across modalities: Identifying corresponding elements in different data types.
Advantages:
- Can effectively model complex interactions between modalities.
- Offers interpretability through attention weights.
Limitations:
- Computationally expensive for large-scale datasets.
- May require careful tuning of attention mechanisms.
3. Hierarchical Approaches
Hierarchical approaches break down the problem into multiple levels, processing information from different modalities at different granularities. This can be achieved through:
- Multi-level fusion: Combining information at different levels of abstraction.
- Hierarchical attention: Applying attention mechanisms at multiple levels.
Advantages:
- Can handle complex and nested structures in data.
- Offers flexibility in modeling relationships between modalities.
Limitations:
- Requires careful design of hierarchical structures.
- May suffer from increased complexity.

Advantages of Multi-Modal RAG Over Traditional Approaches
- Enhanced Understanding: MM-RAG can capture richer and more nuanced information compared to text-only systems.
- Improved Accuracy: By incorporating multiple data sources, MM-RAG can provide more accurate and reliable responses.
- Increased Engagement: Multimodal presentations can be more engaging and informative for users.
- New Applications: MM-RAG opens up new possibilities for applications in various domains, such as education, healthcare, and entertainment.
Limitations of Multi-Modal RAG
- Data Availability: Acquiring large-scale, high-quality multimodal datasets can be challenging.
- Computational Resources: Training and deploying MM-RAG models require significant computational power.
- Model Complexity: Designing effective MM-RAG models can be complex and requires expertise.
- Ethical Considerations: MM-RAG raises ethical concerns related to bias, privacy, and misuse of data.
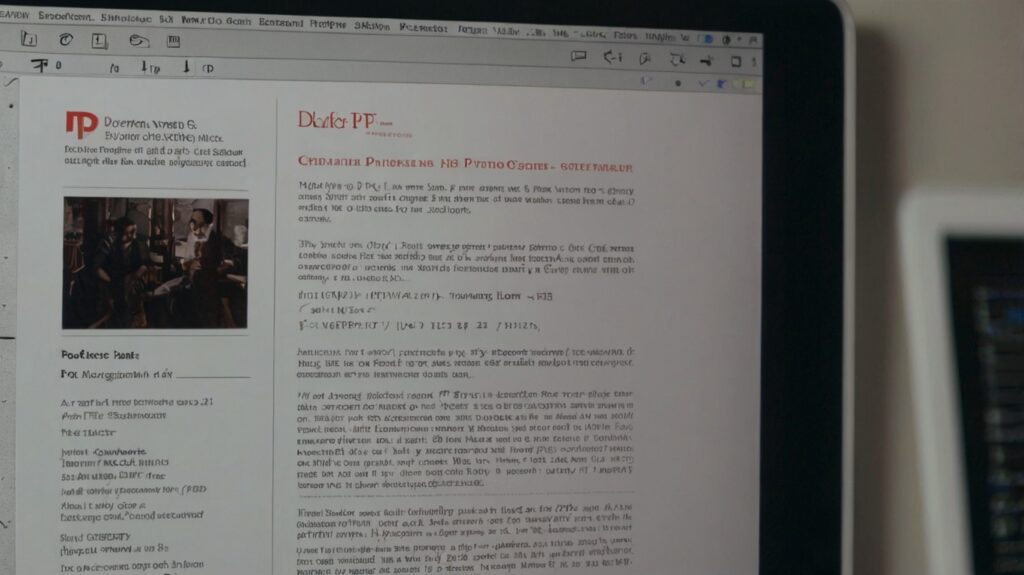
Conclusion
Multi-modal RAG represents a significant advancement in the field of AI, with the potential to revolutionize how we interact with information. By combining the power of retrieval and generation with the ability to process multiple data modalities, MM-RAG systems can provide more comprehensive, accurate, and engaging responses to complex queries.
While there are still challenges to overcome, the potential benefits of MM-RAG are immense. As research and development in this area continue to progress, we can expect to see even more sophisticated and powerful multimodal AI systems emerge in the future.
FAQs (Frequently Asked Questions)
Multi-Modal RAG (MM-RAG) is an advanced form of AI that combines the power of search and generative AI to process and understand various types of data, including text, images, videos, and audio. Unlike traditional RAG systems that focus solely on text, MM-RAG can provide more comprehensive and informative responses to complex queries by leveraging information from multiple sources.
A Multi-Modal RAG system typically consists of two main components:
- Multimodal Retrieval: This involves extracting relevant information from different data types (text, images, videos, etc.) based on the given query.
- Multimodal Generation: This involves using a language model to generate a response by incorporating information from all retrieved modalities.
Multi-Modal RAG offers several advantages over traditional RAG systems, including:
- Enhanced understanding of complex information
- Improved accuracy and reliability of responses
- Increased engagement through multimodal presentations
- Ability to tackle new applications in various domains
While Multi-Modal RAG holds great promise, it also presents certain challenges:
- Difficulty in acquiring large-scale, high-quality multimodal datasets
- High computational requirements for training and deploying models
- Complexity in designing effective multimodal models
- Ethical concerns related to bias, privacy, and misuse of data
Multi-Modal RAG has the potential to revolutionize various industries. Some potential applications include:
- Enhanced search engines that can understand and process visual content
- Intelligent virtual assistants capable of answering questions based on images and videos
- Medical image analysis and diagnosis systems
- Educational tools that provide interactive and engaging learning experiences